Deep Learning for Computer Vision
Explore the field of computer vision using deep learning in this comprehensive course. We cover key areas including image classification, object detection, segmentation, image synthesis, and video analysis. We start with the basics and work our way up to advanced topics such as popular neural network architectures and how to develop your own.
What will I learn?
- Understand what computer vision is and what are the basic concepts of computer vision.
- Refresh your knowledge of deep learning in preparation of learning how to use it for computer vision.
- Learn how to use already finished popular models and how to create new custom ones.
- Complete at least one full capstone project, combining the knowledge gained in this course to solve a complex computer vision problem.
Curriculum
Review of Deep Learning Concepts
Introduction to Computer Vision
Convolutional Neural Networks
Autoencoders, Generative Adversarial Networks (GANs), Transformers and Diffusion Models
Object Recognition and Image Synthesis
Deep Learning and Video Analysis
Capstone Projects
Why Edlitera?
Build the coding, data and AI skills you need, online, on your own schedule. From learning to code as a beginner to mastering cutting-edge data science, machine learning and AI techniques.
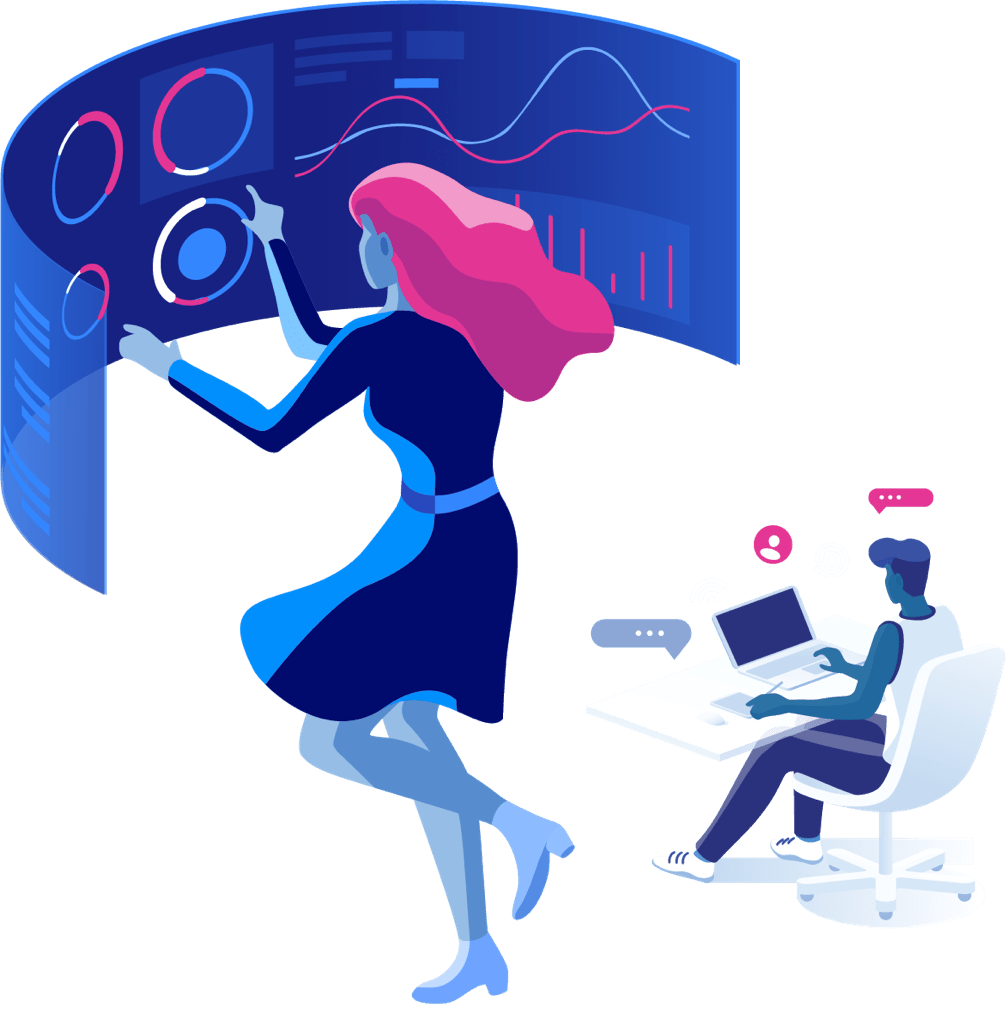
Learning for the real world
Our courses are made with the input and feedback of top teams at Fortune 500 companies in Silicon Valley and on Wall Street.
No-fluff learning
Each minute of each course is packed full of insight, best practices and real-world experience from our expert instructors.
Learn by doing
Start writing code on your computer from Day One. Practice on hundreds of exercises. Apply your skills in mini-projects. Get instant feedback from video solutions.
Complete learning tracks
With over 150 hours of video lectures and hundreds of practice exercises and projects, our learning tracks will help you level up your skills whether you are a novice or an advanced learner.
Course Syllabus
Review of Deep Learning Concepts
- Basics of artificial neural networks
- Basics of Deep Learning in Python
- Stages of a typical Deep Learning project
- Introduction to Transfer Learning
- Advantages, disadvantages and applications of Transfer Learning
Introduction to Computer Vision
- What is Computer Vision?
- Analyzing and processing images for deep learning
- Analyzing and processing videos for deep learning
- Image classification
- Object detection in images
- Object segmentation in images
- Image synthesis
- Video analysis
Convolutional Neural Networks
- Introduction to convolutional neural networks
- Parts of a convolutional neural network
- Convolution and convolutional layers
- Pooling and pooling layers
- Activation functions
- Loss functions
- Standard networks with dense layers
- Fully convolutional Networks
- Applications of convolutional neural networks
- Common applications of CNNs
- Applications of CNNs in the field of computer vision
- Popular CNN architectures
- Using finished architectures over custom architectures
- Basics of the top 10 most popular CNN architectures
- Leveraging the power of transfer learning
Autoencoders, Generative Adversarial Networks (GANs), Transformers and Diffusion Models
- Introduction to Autoencoders
- Parts of an Autoencoder
- Autoencoder input layer, bottleneck, and output layer
- Different types of Autoencoders
- Denoising Autoencoders
- Sparse Autoencoders
- Deep Autoencoders
- Undercomplete Autoencoders
- Convolutional Autoencoders
- Variational Autoencoders
- Applications in the field of computer vision
- Popular Autoencoder architectures
- Introduction to GANs
- Parts of a GAN
- Structure of a typical GAN
- Generator
- Discriminator
- Applications of GANs
- Common Applications of GANs
- Applications in the field of computer vision
- Popular Gan Architectures
- Intro to Transformers
- General architecture of a vanilla Transformers network
- Vision Transformer network (ViT)
- Image classification using Vision Transformers
- Transfer learning with Vision Transformers
- Diffusion models
Object Recognition and Image Synthesis
- Introduction to image classification
- Binary image classification
- Multiclass image classification
- Multilabel image classification
- Introduction to object detection
- Object localization
- How does object detection work?
- Applications of object detection
- Popular architectures of object detection
- Introduction to object segmentation
- How does image segmentation work?
- Semantic segmentation
- Instance segmentation
- Applications of object segmentation
- Popular architectures of object segmentation
- Introduction to image synthesis
- How does image synthesis work?
- Applications of image synthesis
- Using GANs for image synthesis
- Using diffusion models for image synthesis
Deep Learning and Video Analysis
- Introduction to working with videos
- Videos as a type of data
- What is a video?
- Basics of video analysis
- Videos as a data type in deep learning
- Video analysis tasks
- Object tracking
- Action classification
- Neural networks used for analyzing videos
- CNNs and video analysis
- Recurrent networks and video analysis
- Popular architectures
- Specialized architectures
Capstone Projects
- Project 1: Image Segmentation
- Project 2: Video Classification
Frequently Asked Questions
This course is aimed at those with in-depth knowledge of machine learning and deep learning, such as Machine Learning Engineers, Deep Learning Engineers, Software Developer and Computer Vision Engineers.
- Strong Python programming skills are required.
- An understanding of Machine Learning concepts is required.
- An understanding of Deep Learning concepts is required.
- An understanding of basic computer vision concepts is useful, but not necessary.
This course is currently available as a Live Online Instructor-led. This format is available to teams and groups of 3 or more. It consists of live sessions with an expert instructor, which combine lecturing, hands-on exercises to be solved both individually and in pairs, as well as one or two larger projects that require participants to use their newly acquired knowledge to solve real-world problems.
Exercises and projects are chosen to be relevant to the type of problems that your team encounters daily. Our goal is to deliver practical, immediately applicable knowledge, and to empower your team to hit the ground running after each training.
Of course! While we do offer a standard version of this course, we can also work with you to tailor the content such that it fits your team's priorities perfectly.
If you have other questions, or want to find out more about this course or other courses we offer, please email us at contact@edlitera.com.
What people are saying
"I walked into the bootcamp with some basic Python syntax and walked out with a much stronger, contextualized grasp of Python, an understanding of common mistakes, the ability to solve basic coding problems, and confidence in my ability to learn more."
Randi S., Edlitera Student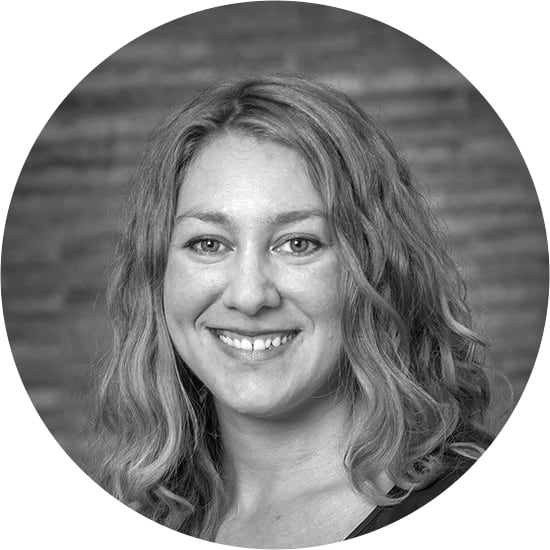
"I wanted to learn Python and be able to process data without being tied and limited by Excel and macros. These classes gave me all the tools to do so and beyond. The materials provided, the engagement of the class by the tutors and their availability to help us were excellent."
Gaston G., Edlitera Student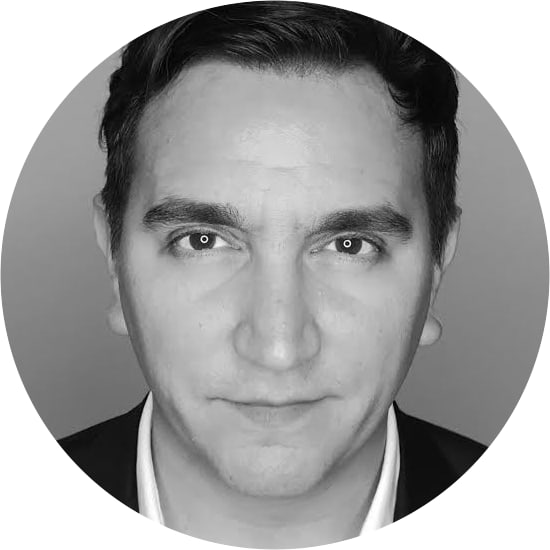
Have a question?
Contact us any time, we’d love to hear from you!